Jul 13, 2022
No image
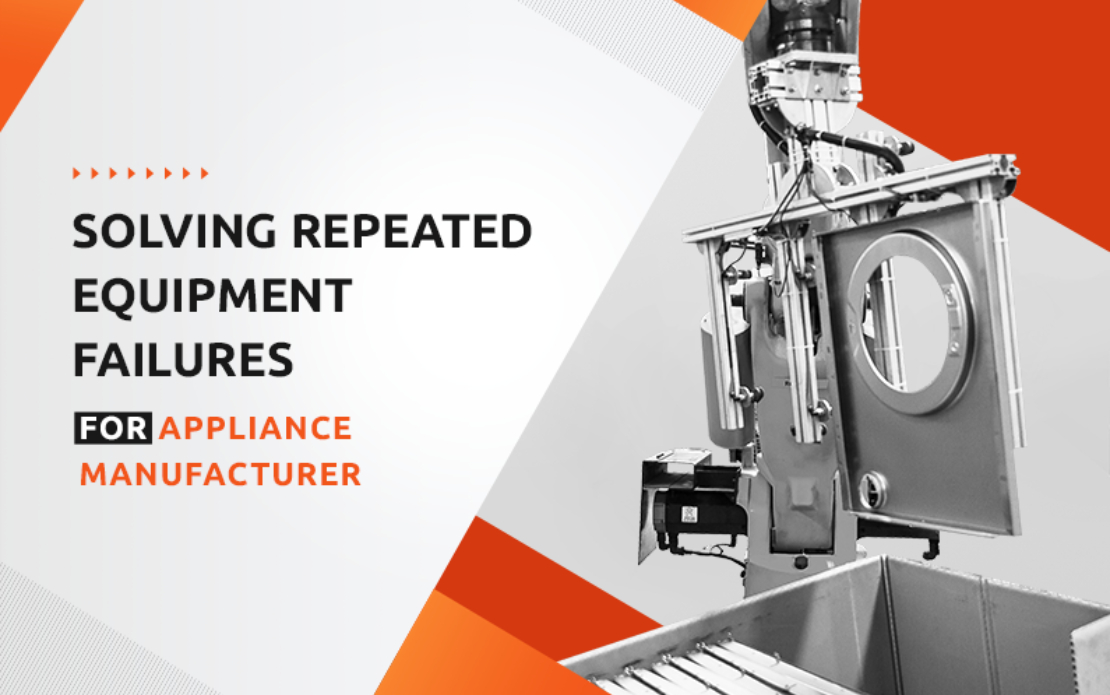
Completed
ML-powered Equipment Failure Prediction Solution for Appliance Manufacturer
$10,000+
4-6 months
United States
2-5
Service categories
Service Lines
Artificial Intelligence
Big Data
Cloud Consulting
Domain focus
Manufacturing
Challenge
Our customer, a global appliance manufacturer, had to deal with repeated equipment failures in their production line. The company wanted to reduce equipment breakdowns, which caused heavy costs and performance inefficiencies in their production.
We’ve built a machine learning solution to predict system failures ahead of time and reduce breakdowns to a minimum acceptable level.
Our customer, a global appliance manufacturer, had to deal with repeated equipment failures in their production line. The company wanted to reduce equipment breakdowns, which caused heavy costs and performance inefficiencies in their production.
We’ve built a machine learning solution to predict system failures ahead of time and reduce breakdowns to a minimum acceptable level.
Solution
Our team built the scalable data processing unit on top of Dask and deployed it to the AWS Fargate cluster. This allowed to process and analyze huge historical and real-time datasets collected from IoT/IIoT sensors. Next, we solved the sparsity data problem by converting data into a different format. Our data scientists needed to eliminate data sparsity to avoid too many gaps present in the collected data, which affected its quality. The team performed feature engineering for categorical, numerical, and timestamp data to improve the future model results based on the available historical data. They experimented with different algorithms and chose the Extreme Gradient Boosting classifier for modeling. After they defined policies and conducted education of factory personnel
Our team built the scalable data processing unit on top of Dask and deployed it to the AWS Fargate cluster. This allowed to process and analyze huge historical and real-time datasets collected from IoT/IIoT sensors. Next, we solved the sparsity data problem by converting data into a different format. Our data scientists needed to eliminate data sparsity to avoid too many gaps present in the collected data, which affected its quality. The team performed feature engineering for categorical, numerical, and timestamp data to improve the future model results based on the available historical data. They experimented with different algorithms and chose the Extreme Gradient Boosting classifier for modeling. After they defined policies and conducted education of factory personnel
Results
As a result, our machine learning model showed over 90% accuracy in equipment failure prediction. So, the manufacturer could reduce their maintenance cost by 5% as well as optimize the overall performance of their production line.
As a result, our machine learning model showed over 90% accuracy in equipment failure prediction. So, the manufacturer could reduce their maintenance cost by 5% as well as optimize the overall performance of their production line.