Dec 19, 2023
No image
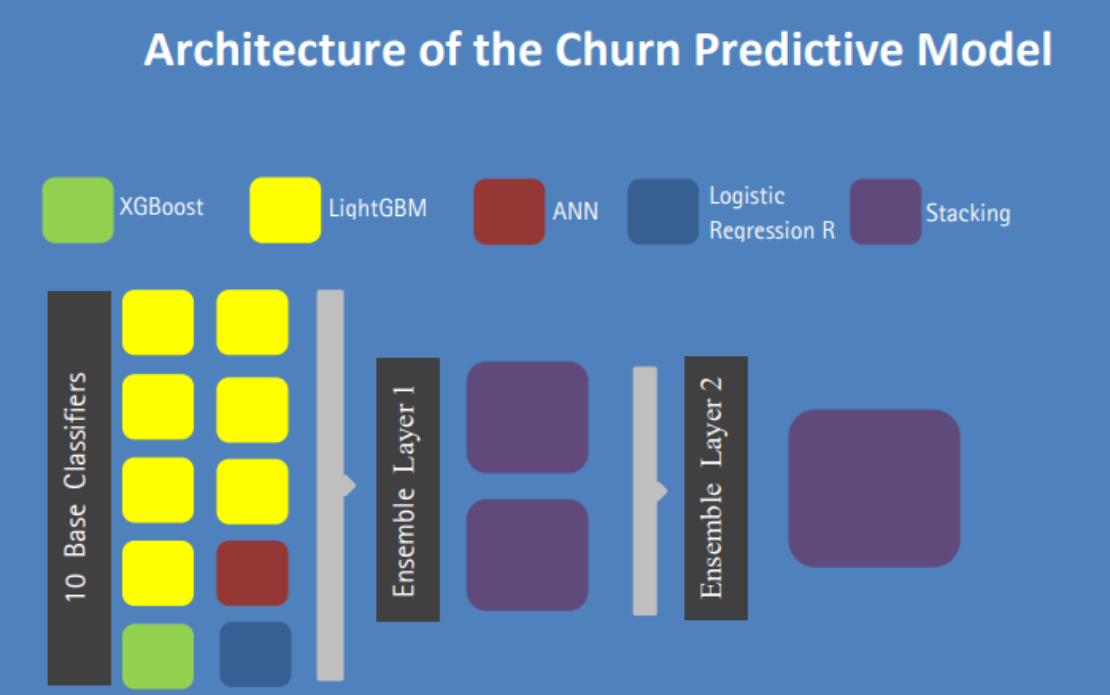
Completed
Customer Churn Predictive Model
$25,000+
4-6 months
United States, San Francisco
6-9
Service categories
Service Lines
Big Data
Domain focus
Consumer Products & Services
Subcategories
Big Data
Marketing Analytics
Challenge
Data Complexity: Dealing with 11 years of diverse data, including membership details, transactions, and user logs, posed a challenge in extracting meaningful insights.
Feature Engineering: Creating relevant features to capture user behavior and interactions required a thorough understanding of the business context and effective categorization.
Class Imbalance: Addressing the class imbalance issue in predicting customer churn was crucial for model performance and reliability.
Model Diversity: Ensuring diverse and independent base models for the ensemble approach to enhance predictive accuracy and robustness.
Data Complexity: Dealing with 11 years of diverse data, including membership details, transactions, and user logs, posed a challenge in extracting meaningful insights.
Feature Engineering: Creating relevant features to capture user behavior and interactions required a thorough understanding of the business context and effective categorization.
Class Imbalance: Addressing the class imbalance issue in predicting customer churn was crucial for model performance and reliability.
Model Diversity: Ensuring diverse and independent base models for the ensemble approach to enhance predictive accuracy and robustness.
Solution
Advanced EDA: Exploratory Data Analysis provided insights into user registration trends and identified key factors influencing churn, guiding feature selection and model development.
Feature Categorization: Effective feature engineering categorized data into Membership, User Log, and Transactional features, enabling a nuanced understanding of user behavior and interactions.
Ensemble Modeling: Utilizing an ensemble approach with ten diverse base models from different feature sets mitigated class imbalance issues, enhancing model performance.
Time-Dependent Analysis: Implementing time windows in feature generation ensured capturing variations in user behavior, making predictions more dynamic and reflective of temporal patterns.
Advanced EDA: Exploratory Data Analysis provided insights into user registration trends and identified key factors influencing churn, guiding feature selection and model development.
Feature Categorization: Effective feature engineering categorized data into Membership, User Log, and Transactional features, enabling a nuanced understanding of user behavior and interactions.
Ensemble Modeling: Utilizing an ensemble approach with ten diverse base models from different feature sets mitigated class imbalance issues, enhancing model performance.
Time-Dependent Analysis: Implementing time windows in feature generation ensured capturing variations in user behavior, making predictions more dynamic and reflective of temporal patterns.
Results
Auto-renew and cancellation ratios emerged as key churn indicators, highlighting the pivotal role of transactional attributes. The final ensemble model excelled with a remarkable 96% accuracy and 86.5% F1-score, surpassing individual models and showcasing robustness. Transactional features, encompassing discounts, payment types, and subscription plans, outweighed user activity in predicting churn. In a saturated market, where quality is uniform among service providers, user activity became an unreliable predictor. The findings underscore the imperative for transaction-focused retention strategies in such competitive landscapes.
Auto-renew and cancellation ratios emerged as key churn indicators, highlighting the pivotal role of transactional attributes. The final ensemble model excelled with a remarkable 96% accuracy and 86.5% F1-score, surpassing individual models and showcasing robustness. Transactional features, encompassing discounts, payment types, and subscription plans, outweighed user activity in predicting churn. In a saturated market, where quality is uniform among service providers, user activity became an unreliable predictor. The findings underscore the imperative for transaction-focused retention strategies in such competitive landscapes.