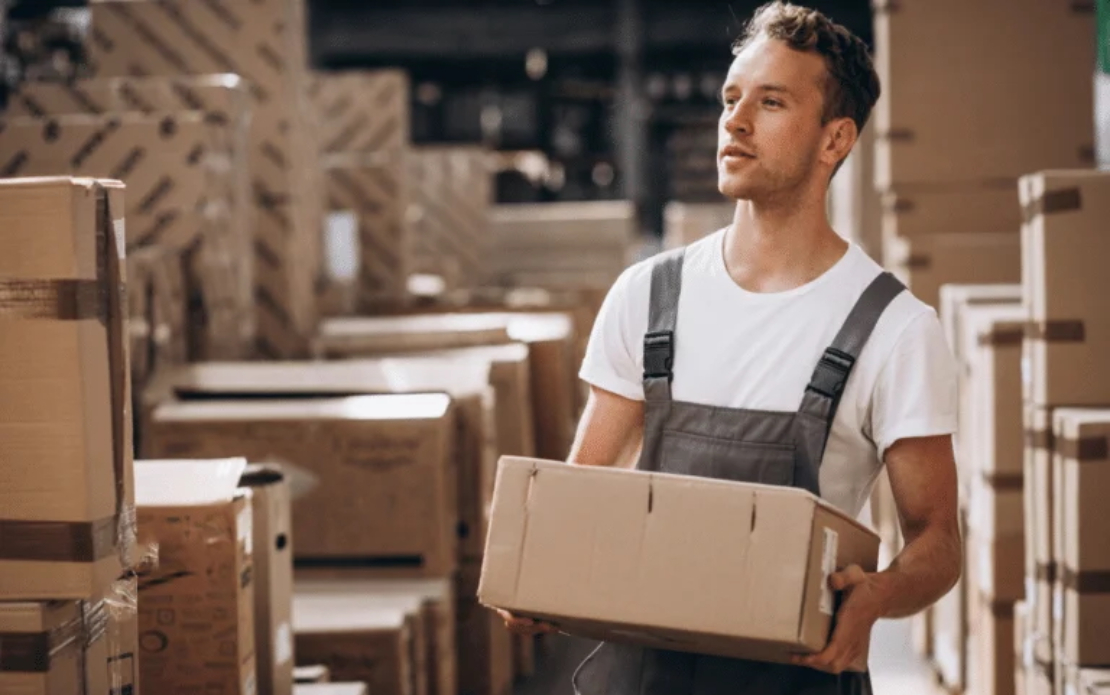
Next-Level Data Platform for Amazon FBA Sellers
Challenge
Back in 2018, the company raised seed funding from family and friends and began building a team of experts in e-commerce, marketing, and operations. Several successful cases have confirmed market opportunities and business models: Investments in Amazon FBA brands, retailers, and marketplaces.
Business stakeholders require more analytical tools and market data in their arsenal to scale successful strategies to the next retailers and brands, whose number is measured in the hundreds of millions.
Collect big data across the industry, analyze data for each Amazon FBA seller, and build data science prediction model in order to determine the potential for attracting investment and best practices. Therefore, bringing data platform and analytical tools to the next level became critical challenge to enable further business growth.
The biggest challenge for Upstaff was to assemble a team of data science and engineering experts, who would build the platform from scratch:
The data platform itself (across data science teams, data engineers and database administrators)
Architect and design a full data stack and infrastructure that will eventually become an e-commerce business with data scraping and analytics with scalability, fault tolerance and security in mind.
Back in 2018, the company raised seed funding from family and friends and began building a team of experts in e-commerce, marketing, and operations. Several successful cases have confirmed market opportunities and business models: Investments in Amazon FBA brands, retailers, and marketplaces.
Business stakeholders require more analytical tools and market data in their arsenal to scale successful strategies to the next retailers and brands, whose number is measured in the hundreds of millions.
Collect big data across the industry, analyze data for each Amazon FBA seller, and build data science prediction model in order to determine the potential for attracting investment and best practices. Therefore, bringing data platform and analytical tools to the next level became critical challenge to enable further business growth.
The biggest challenge for Upstaff was to assemble a team of data science and engineering experts, who would build the platform from scratch:
The data platform itself (across data science teams, data engineers and database administrators)
Architect and design a full data stack and infrastructure that will eventually become an e-commerce business with data scraping and analytics with scalability, fault tolerance and security in mind.
Solution
Quick start with one data architect expert for Data Lake & MVP sooner evolved into a full scale data science, data engineering & quality team:
Data lake, data architecture, and data quality for all incoming data pipelines (scrapers, AWS APIs, raw DBs),
Dashboards & reporting for stakeholders and decision-makers.
Over a decade, the team grew to 14 people:
- Data Engineering Team
(Data architecture & Engineering tasks, ETLs, data pipelines, Infrastructure, third-party data sources integrations, data scraping tools) - Data Quality Automation Team
(Data integrity, persistency & quality, staging according to the requirements, transformation for BI tools) - Business Analyst
- Business Intelligence and Analytics Team
(Dashboards & Reporting)
Technology Stack
The choice of engineering technology lay between Scala and Python. The latter was prioritized to enable future team scaling (Scala engineers are rarer).
Technology stack included:
- Python for scripting and data processing
- Amazon AWS Redshift, Snowflake for Data Warehousing
- AWS EMR as the core data platform
- Apache Spark with Python and Scala for data processing
- Kubernetes is one of the core infrastructure
- Power BI, Airflow for dashboards and reporting
Data Security & Privacy
All engineers are certified in data analytics and throughout the process of building data systems we follow established best practices: AWS safety rules, VPN, Private/Public subnets, production and development environments in different AWS accounts, and AWS Secrets Manager.
Project stack
- Python
- Amazon Web Services (AWS)
- AWS Redshift
- Snowflake
- Data Pipelines (ETL)
- PySpark
- Apache Spark
- Kubernetes (K8s)
- Microsoft Power BI
- Apache Airflow
Quick start with one data architect expert for Data Lake & MVP sooner evolved into a full scale data science, data engineering & quality team:
Data lake, data architecture, and data quality for all incoming data pipelines (scrapers, AWS APIs, raw DBs),
Dashboards & reporting for stakeholders and decision-makers.
Over a decade, the team grew to 14 people:
- Data Engineering Team
(Data architecture & Engineering tasks, ETLs, data pipelines, Infrastructure, third-party data sources integrations, data scraping tools) - Data Quality Automation Team
(Data integrity, persistency & quality, staging according to the requirements, transformation for BI tools) - Business Analyst
- Business Intelligence and Analytics Team
(Dashboards & Reporting)
Technology Stack
The choice of engineering technology lay between Scala and Python. The latter was prioritized to enable future team scaling (Scala engineers are rarer).
Technology stack included:
- Python for scripting and data processing
- Amazon AWS Redshift, Snowflake for Data Warehousing
- AWS EMR as the core data platform
- Apache Spark with Python and Scala for data processing
- Kubernetes is one of the core infrastructure
- Power BI, Airflow for dashboards and reporting
Data Security & Privacy
All engineers are certified in data analytics and throughout the process of building data systems we follow established best practices: AWS safety rules, VPN, Private/Public subnets, production and development environments in different AWS accounts, and AWS Secrets Manager.
Project stack
- Python
- Amazon Web Services (AWS)
- AWS Redshift
- Snowflake
- Data Pipelines (ETL)
- PySpark
- Apache Spark
- Kubernetes (K8s)
- Microsoft Power BI
- Apache Airflow
Results
Outcome
- Customer brands are sold across more than 150 retailers and marketplaces.
- As a leading aggregator of Amazon.com Inc. products, the customer raised more than $1 billion in a private funding round led by a technology investor. The company’s evaluation exceeded $1 billion and became a unicorn.
- 90% of the company’s investment decisions are made on the basis of analytics and data from the platform, which made it an integral tool for daily work on a competitive edge in the boundless ocean of information, marketplaces, and Amazon FBA business sellers.
Outcome
- Customer brands are sold across more than 150 retailers and marketplaces.
- As a leading aggregator of Amazon.com Inc. products, the customer raised more than $1 billion in a private funding round led by a technology investor. The company’s evaluation exceeded $1 billion and became a unicorn.
- 90% of the company’s investment decisions are made on the basis of analytics and data from the platform, which made it an integral tool for daily work on a competitive edge in the boundless ocean of information, marketplaces, and Amazon FBA business sellers.