May 29, 2023
No image
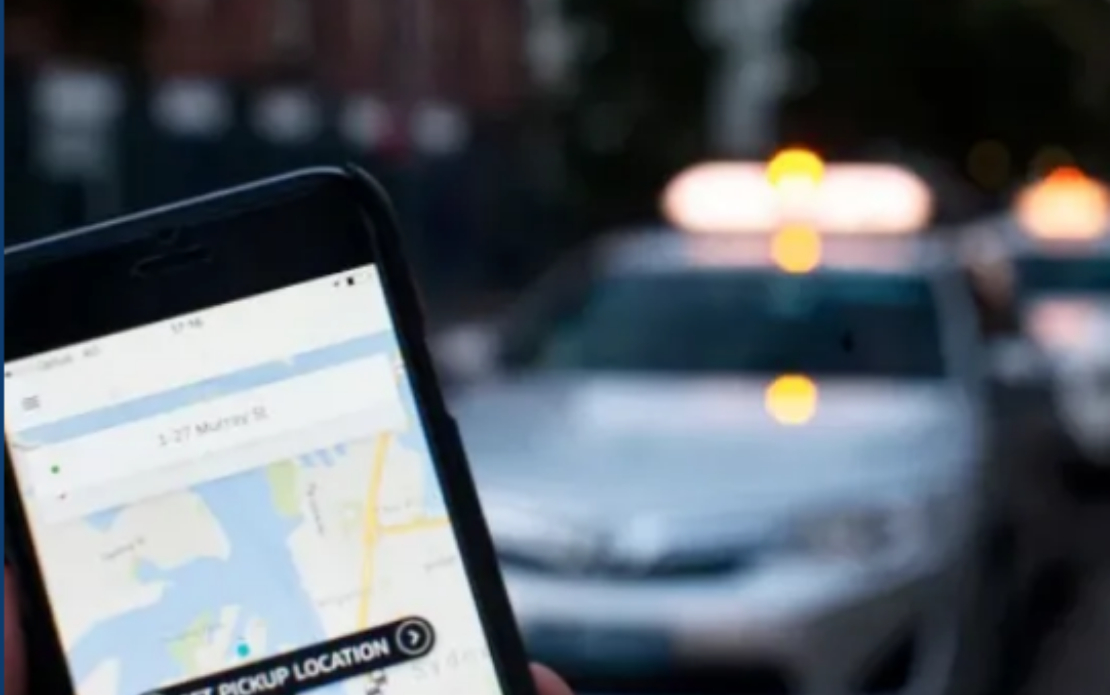
Completed
Ride Sharing App: Revenue Up 21% via Predictive AI Modeling
$10,000+
2-3 months
United States
2-5
Service categories
Service Lines
Artificial Intelligence
Domain focus
Transportation & Logistics
Subcategories
Artificial Intelligence
Machine Learning
Challenge
The client is a carpooling and car ride sharing app. App drivers face challenges knowing where to position their vehicles to find waiting passengers, and passengers express frustration trying to find an available driver.
The client is a carpooling and car ride sharing app. App drivers face challenges knowing where to position their vehicles to find waiting passengers, and passengers express frustration trying to find an available driver.
Solution
Vacon’s team of data scientists and developers employ the latest in geo-point data tracking and devise a novel solution through predictive modeling.
How it works:
A clustering algorithm records geo-points and rides at a given time of the day and groups trips into regions.
Flask API is the layer built on top of the algorithm and retrieves geo-location based on driver’s current position.
Geo-points are retrieved using OSMNX framework in python and parsed into arrays that can be processed via Machine Learning Libraries. Libraries retrieve roads and distances based on car mode of transportation.
The ride sharing app suggests with statistical confidence (from machine learning) where drivers which route and destination to increase the likelihood of finding waiting passengers.
Vacon’s team of data scientists and developers employ the latest in geo-point data tracking and devise a novel solution through predictive modeling.
How it works:
A clustering algorithm records geo-points and rides at a given time of the day and groups trips into regions.
Flask API is the layer built on top of the algorithm and retrieves geo-location based on driver’s current position.
Geo-points are retrieved using OSMNX framework in python and parsed into arrays that can be processed via Machine Learning Libraries. Libraries retrieve roads and distances based on car mode of transportation.
The ride sharing app suggests with statistical confidence (from machine learning) where drivers which route and destination to increase the likelihood of finding waiting passengers.
Results
80% predictive accuracy meant drivers booked an average of 32% more passenger rides.
Reduced passenger waiting time by 7 minutes.
Anecdotal results: less carbon emissions, wasted fuel, vehicle running costs, and less traffic congestion (the right vehicle, in the right place, at the right time).
21% more revenue booked by ride sharing app due to increased productivity from route efficiencies.
80% predictive accuracy meant drivers booked an average of 32% more passenger rides.
Reduced passenger waiting time by 7 minutes.
Anecdotal results: less carbon emissions, wasted fuel, vehicle running costs, and less traffic congestion (the right vehicle, in the right place, at the right time).
21% more revenue booked by ride sharing app due to increased productivity from route efficiencies.