How to Build an AI Image Recognition Platform for Healthcare
Medical staff has many clinical challenges and developers have many solutions, if you put the two of them together, you’ve got a great opportunity for innovation. In this article, we describe how modern AI technologies help leverage the experience and skills of doctors to help more patients, detect diseases at earlier stages and set more correct diagnoses.
Image recognition represents a set of methods for detecting and analyzing images to enable the automation of a specific task. This technology is capable of identifying places, people, objects, and many other types of elements within an image, and drawing conclusions by analyzing it.
The use of image visualization and recognition software in medical diagnostics started over 20 years ago with Computer Vision, which worked very well with hard tissue analysis, like X-rays. However, computer vision never allowed creating a reliable diagnostic system that would exclude the doctor from setting a diagnosis. A new performance limit was reached with the introduction of Deep Learning (DL) and Convolutional Neural Networks (CNNs) which was a game-changer for the Computer Vision industry.

What is a Medical Imaging AI Platform for healthcare?
An Artificial Intelligence platform is a system created to perform more accurately and logically than regular technical frameworks. In medical imaging, AI technologies can be used to detect cancer, cardiovascular diseases, respiratory diseases, retinal diseases and neurodegenerative diseases. Machine learning-based approaches allow doctors to detect abnormalities in imaging data and adjust or accelerate the treatment.
Such platforms are created by Data Scientists and Medical Subject Matter Experts who train the machine learning models, create algorithms and pipelines to analyze 2D and 3D images made with various types of medical equipment.
The essential part of a Medical Imaging AI Platform is a dataset, which is a universal unit allowing engineers to communicate properly with subject matter experts (i.e., doctors). A dataset contains thousands of images of the same condition or pathology in as many variations as possible. It should contain all known cases and forms of the pathology, as well as marginal cases.
What are the advantages of the Medical Imaging AI Platform for patients and healthcare organizations?
The main benefits for patients are:
- Exclude the possibility of a wrong diagnosis. AI models help avoid cases when the doctor gets tired, distracted or is less experienced. Through big datasets, AI can provide patients access to the knowledge and experience of hundreds of doctors instead of just one who is treating them.
- Reduce the time for setting a diagnosis. AI models assist doctors to find abnormalities faster and more accurately
- Detect the disease earlier. An AI-powered model is able to detect formations that are smaller in size, which draws doctor’s attention to the regions of interest
Benefits for healthcare organizations:
- Improve the economy, efficiency and effectiveness of clinical processes.
- Support clinical decision making.
- Enable physicians to practice at the top of their license by treating complex cases instead of doing routine work.
- Make healthcare more affordable and accessible to more patients.
- Scale doctors’ expertise required for mass screenings
Why create a Medical Imaging AI Platform in 2021. Opportunities
IDTechEx predicts the growth of AI-powered image-based medical diagnostics. In value terms, AI-enabled image-based medical diagnostics will reach $3 billion by 2030 across five segments including cancer and CVD as well as respiratory, retinal and neurodegenerative diseases.
In terms of trends, AI technology will be developing to match and exceed the abilities of a human in accuracy, sensitivity, and specificity. The technical threshold for the automation of diagnostic tasks has already been reached, which lays the foundation for commercial growth in the short and long term.
With more and more patient data accumulating, there will be more opportunities to create better datasets that would be more and more accurate in detecting abnormalities. This will require increasing the computational resources, so medical organizations will tend to use cloud solutions except for those who prefer to keep their data inside. They will be using their own data centers while increasing their capacities.
Companies will need to make a strong focus on data acquisition policies at the heart of the product development. Partnerships and customer relations will be equally important. This will protect their business, acting as a barrier to entry for newcomers.
Medical Imaging AI Platform development stages
There is no unified solution that would fit all purposes. The specific product development workflow depends on the organization, the problem that needs to be solved and the goal of the project. However, we can define some stages that each machine learning project will undergo.
1. Define project objectives
- Specify business problem
- Acquire subject matter expertise
- Define the unit of analysis and prediction target
- Prioritize modelling criteria
- Consider risks and success criteria
2. Acquire and Explore Data
- Find Appropriate data
- Merge data into a single database
- Conduct exploratory data analysis
- Find and remove any target leakage
- Launch feature engineering
3. Model data
- Select variables
- Build candidate models
- Validate and select a model
4. Interpret & communicate
- Interpret model
- Communicate model insight
5. Implement, Document & Maintain
- Set up a batch or API prediction system
- Start document modelling process for reproducibility
- Create model monitoring and maintenance plan
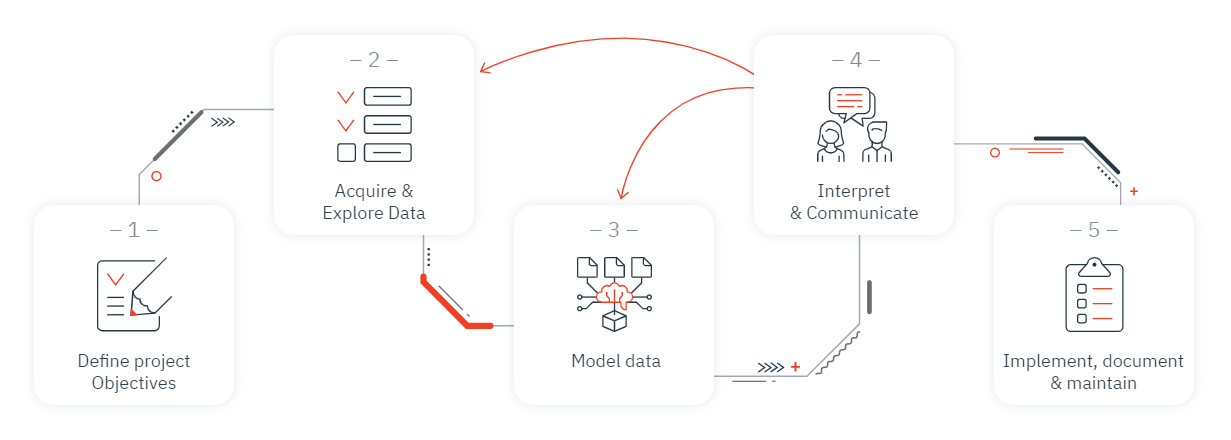
Some problems you may face
1. Dataset quality
As a rule, open datasets available to the public are made in ideal conditions. They were initially meant for research purposes, so they basically contain perfect images with good resolution that had been made properly. Such perfect images are very rare in real life. As experience shows, pictures taken in the real clinical workflow are usually far from perfect and might be blurry, lack focus or made improperly. For an AI model to work accurately in real-life conditions, we need to train the model on real-life examples.
2. Overfitting
Data engineers should always have at least 2 or 3 datasets on hand for their AI image recognition project. The first and the largest one is used to train the model. If the model is trained incorrectly, this may lead to overfitting, which is a common problem in the machine learning project pipeline. It means that the model learns not to distinguish between the images containing the disease or not. Instead, it will remember the exact pictures, which is the wrong logic. In this case, the other dataset will have to be used to train the model over again.
3. Marginal cases
In case of bone fractures, it is usually relatively easy to set a diagnosis. The fracture is either there or not. With some conditions, like diabetic retinopathy, the doctors’ opinions may differ on whether the patient has the condition or not. This is a huge problem for medical imaging nowadays that is yet to be solved. The same image can be put in one category by one expert and the other category by the other. Your machine learning model may end up setting diagnoses that the doctors don’t agree with. The solution would be training the model in such a way when 6 doctors out of 10 would agree with the diagnosis established.
4. Bias
Due to differences in data policies of various organizations, a lot of the data used today to train AI algorithms for diagnosing diseases might record inequalities. Research shows that almost all eye disease datasets come from patients in North America, Europe, and China, meaning eye disease-diagnosing algorithms are less certain to work well for other racial and/or geographical groups. This would involve significant issues if such models are used in underrepresented regions or countries.
Conclusion
At present, many diseases can be identified at an early stage with the help of Artificial Intelligence, helping to start earlier treatment. This means a better quality of life for patients and saving numerous lives. The whole medical sphere is undergoing digital transformation at an unprecedented speed. The tendency is to exclude highly qualified personnel from the process of routine screening of diseases and setting diagnoses. This serves to the benefit of both doctors and patients: the time and resources of doctors are freed in favour of actually treating patients.
The trend is to raise the AI complexity beyond trivial object detection. Today, ML algorithms match the abilities of humans, but they already can complete the same tasks faster and/or better. In the future, with more digitization of patient data, we can expect more data fusion, which will probably enable AI to offer insights beyond human capability. This could be a game-changer.