Top 5 Trends in Ethical AI Development
Multiple cases of racism, sexism, and other kinds of biases in algorithms showcased by the media have finally made the industry realize ― adherence to AI ethics principles is a must for AI development.
Ethical AI development is a critical discipline that questions ideas and beliefs that we take for granted. It’s a new field but it has made new approaches to AI development emerge. In this post, let’s talk about the ethical AI development trends that you need to keep an eye on in 2021.
Why is AI ethics necessary?

In the past few years, AI started to attract a lot of negative attention from the press for its weird and biased conclusions. What are the reasons behind an AI algorithm classifying all males under 25 as reckless drivers or seeing all women as unsuitable to work for Amazon?
It turned out that implicit biases of development teams were transported to the datasets. For example, a Google development team that consisted almost exclusively of white men didn’t consider including photos of people of color in their training datasets. As a result, their face recognition algorithm didn’t understand that non-white people are also people and classified them as gorillas or simply didn’t see them!
Are you eager to live in a world where algorithms are biased like humans? After all, artificial intelligence is now used in systems responsible for medical diagnosis and treatment, criminal risk assessment, and university applicant scoring. AI has a huge impact on human lives, and it’s our mission to not let it be harmful.
Trends in ethical AI development that everyone should know
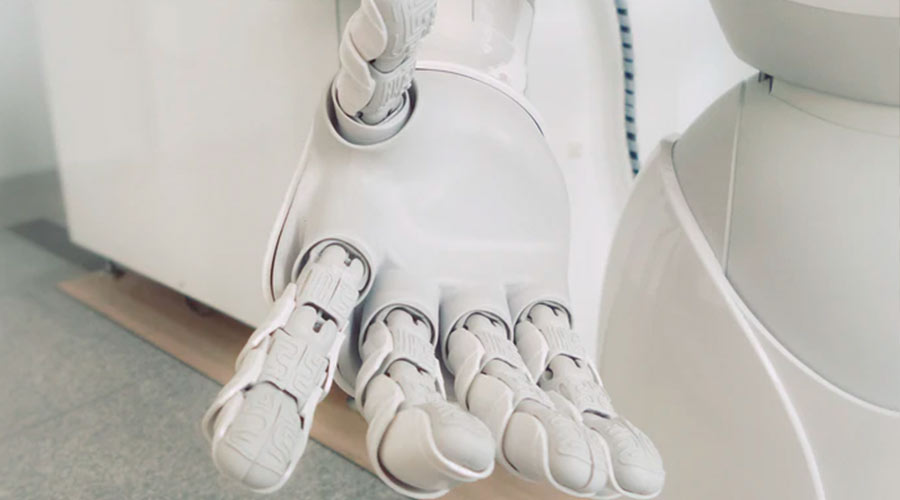
How can we make algorithms we have safer for humanity? Let’s find out.
Human agency and oversight
Artificial intelligence is a technology that is usually judged by its ability to act autonomously. Think about the GPT-3 model that can write anything from code to fake news or Deep Dream Generator that generates art after you press a button.
Creating a fully autonomous system that learns about the environment and improves by itself has always been an interesting challenge for developers. In a way, AI is the embodiment of a dream where machines do all the work and humans enjoy life engaging in arts and hobbies.
However, AI has received a lot of criticism in the last few years in socially sensitive areas. Criminal risk assessment systems were blamed to be racist, and hiring algorithms — sexist. The use of AI in many areas is now being revised, for example, in the military where AI robots called ‘war machines’ are often used. These machines vary in the level of autonomy, but the most advanced ones can make decisions about opening fire without involving a human operator at all.
It is obvious that the initial motives to create AI algorithms that would provide military service were good: robots are much more dependable than humans. In addition, bringing robots to the battlefield would allow reducing the number of war crimes, including sexual violence against women and children.
However, there are so many risks involved too:
- AI is ruthless. Human soldiers have mercy over those who surrender or aren't capable of doing any harm. There are many cases when soldiers from opposing sides help each other if they are heavily wounded. How will we explain to the machine that the enemy is not an enemy anymore?
- AI can be easily tricked. AI is easy to fool. For example, white flags are often a sign of surrender on the battlefield. However, opponents might use white flags on purpose to fool the machine and get closer to it to destroy it.
- AI is confused by chaos. On the battlefield, it’s hard to distinguish between comrades and opponents. Cases of friendly fire are even more likely to happen when AI is involved because smoke, explosions, and fast movements of the soldiers impede them from reacting timely.
Considering all these issues, full automation in the case of war machines is ethically impossible. That is why the trend of today is to move from the human-out-of-the-loop framework (where a machine is fully autonomous) to the human-on-the-loop mechanism. Once there is an extraordinary situation and AI feels confused, a human operator has a chance to interfere and make the right decision.
This tendency is adopted in other areas of life as well, for example, in self-driving cars. Even the most cutting-edge models of Tesla still imply that there is a human driver who monitors the situation on the road at all times.
Technical robustness and safety

AI systems are ubiquitous, and quite often they make very important decisions about our lives. Therefore, when AI fails, the consequences may be tremendous.
In 2013, Syrian hackers got access to the Associated Press Twitter account and posted information about explosions in the White House. Twitter algorithm rated this tweet as reliable because Associated Press had been considered as a reliable source before. Because of that, the Dow Jones Industrial Average dropped by $136 billion dollars. A human would do some fact-checking in other resources before believing this information, but present machine learning systems are not good at identifying sudden changes. They don’t handle the situations of poisoning well because they don’t understand the context.
One of the main focuses of researchers today is to try to improve anomaly detection techniques and overall understanding of context. Without being technologically robust, AI cannot be truly trustworthy.
Transparency
A machine learning model can perform poorly for many reasons. The training data could be badly preprocessed. The hyperparameters of the model could be chosen wrongly. Maybe ML engineers who were working on the model have introduced harmful algorithmic logic on purpose or the behavior of the program has been changed due to adversarial attacks. It is also possible that the model is overfit or underfit, and that is the reason why the results are unreliable.
A lot of things can go wrong. And it’s hard to uncover the source of the problem, since most often the errors are evident only during running time. In the meanwhile, an error can cost a lot both in financial and reputational terms.
That is why AI actions should be tracked, and AI decisions should be subject to explainability. Different legal regulations are implemented in different countries to promote model transparency. The main limitation here is that the majority of ML algorithms are proprietary. The company has legal reasons not to disclose their decision-making process because it is their intellectual property. In such cases, a thorough internal audit should be implemented.
Accountability
Accountability is closely connected to transparency. AI should be able to tell why it took certain actions. Moreover, this information should be made public when applicable. For example, if an AI system is used for criminal risk assessment, the defendant has the right to know how the score will affect his sentence and based on what criteria the decision was made. This approach would help to avoid outrageous cases of unspoken racism that ProPublica recently wrote about in their research dedicated to biases in the USA risk assessment systems.
Diversity and fairness

We all have heard that fairness and diversity are good and discrimination is bad. While the majority of us don't consider ourselves to be racist or sexist, the truth is that many of us have various cognitive biases. They hide very well, and we are profoundly convinced that we treat other people as they deserve to be treated. If you would like to make an experiment go to the Moral Machine website. It makes you solve moral dilemmas as if you were in charge of a self-driving car with broken brakes. Who deserves to die: a businessman or a homeless person? An old respectable lady or a young criminal? You will learn a lot about yourself.
The thing is that AI algorithms do have to solve such problems daily. In 2018, Amazon attempted to use an AI hiring algorithm. Seems like a great solution to promote diversity? In reality, the algorithm considered all female applicants to be a bad fit for any position because historically in the company they were occupied by men. In the end, they couldn’t fix the error and had to dismiss an initiative that cost millions of dollars. To prevent such errors in the products, tech companies should promote diversity in the workplace and raise awareness about sensitive issues.
What trends do you find the most important in ethical AI development? Would you implement AI ethics in your company?