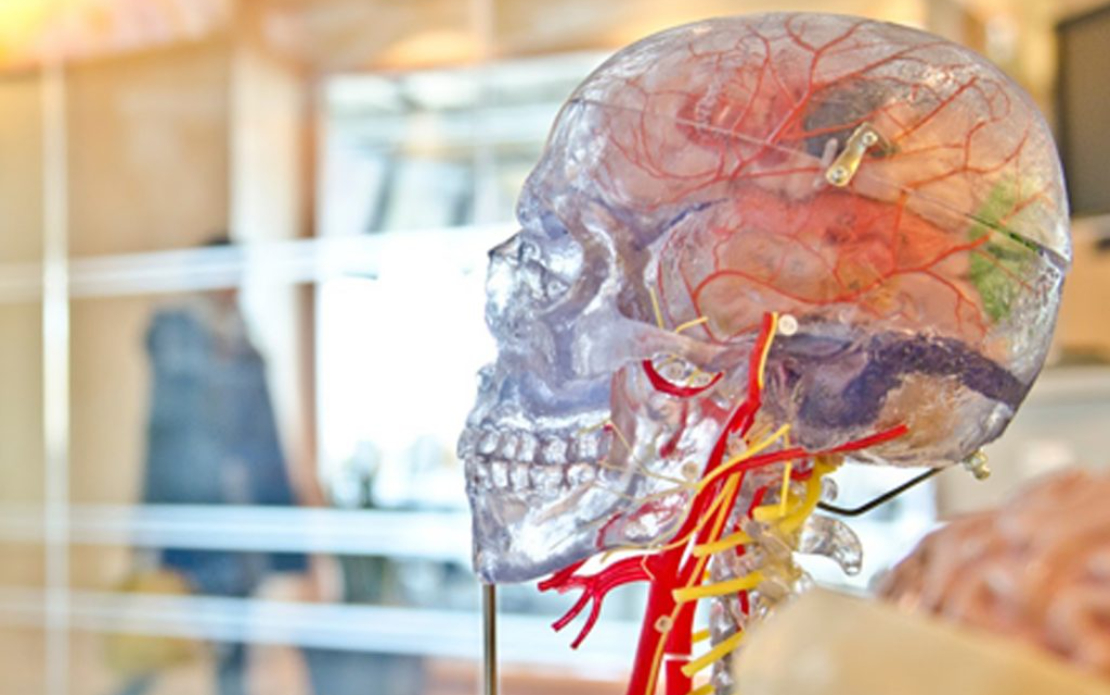
Challenge
Since epilepsy is a severe disease, sometimes doctors need a second opinion to decide on the treatment type that would be the most fitting and safe for a particular type of seizure.
In order to diagnose DRE, a doctor should try various medications first and only after a certain amount of time, which usually takes around 3 years, a patient can be diagnosed with DRE.
Specializing in epilepsy treatment, our client wanted to research ways to help doctors identify drug-resistant epilepsy cases faster – around 1 year, which in its turn could allow patients to get a better quality of life.
Since epilepsy is a severe disease, sometimes doctors need a second opinion to decide on the treatment type that would be the most fitting and safe for a particular type of seizure.
In order to diagnose DRE, a doctor should try various medications first and only after a certain amount of time, which usually takes around 3 years, a patient can be diagnosed with DRE.
Specializing in epilepsy treatment, our client wanted to research ways to help doctors identify drug-resistant epilepsy cases faster – around 1 year, which in its turn could allow patients to get a better quality of life.
Solution
The solution made by our R&D team allows predicting drug or non-drug resistant epilepsy with high accuracy (82% at the moment) for a patient. In situations where a patient has nonDRE, they could try experimental treatment methods. The solution works as a service that can make a prediction for any patient at a doctor’s request.
The solution made by our R&D team allows predicting drug or non-drug resistant epilepsy with high accuracy (82% at the moment) for a patient. In situations where a patient has nonDRE, they could try experimental treatment methods. The solution works as a service that can make a prediction for any patient at a doctor’s request.
Results
We had daily calls with the US-based part of the team. Data preparation As input, we used the data of about 450 thousand patients. It included general information, ICD codes, information about treatment and doses. In an experimental way, we cleared the data. In the next steps, we used raw data for our model. ML model First, we tried different methods and models. We started with the most difficult ones and continued with more common ones. Also, we applied NLP techniques. Integration This model was integrated with Symfony Health to work as a service with access to patient data. It gives a prediction to the doctor before the patient’s visit.
We had daily calls with the US-based part of the team. Data preparation As input, we used the data of about 450 thousand patients. It included general information, ICD codes, information about treatment and doses. In an experimental way, we cleared the data. In the next steps, we used raw data for our model. ML model First, we tried different methods and models. We started with the most difficult ones and continued with more common ones. Also, we applied NLP techniques. Integration This model was integrated with Symfony Health to work as a service with access to patient data. It gives a prediction to the doctor before the patient’s visit.